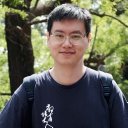
Dr. Yizhou Fan joined the FLoRA project in July 2019 and worked as a post-doc researcher in the School of Informatics at the University of Edinburgh. At the heart of his research program is analytics of self-regulated learning. Specifically, in the FLoRA project, he looked at the novel methodological approaches for the measurement of self-regulated learning through the integration and analysis of multichannel data (e.g., log data, eye-tracking, mouse movements, think-alouds). He then aimed to make use of these data to provide personalized scaffolds and feedback that can be offered to the learners in real-time to enhance their decision making and performance. He published three Journal articles and one conference paper based on the FLoRA project (see below). In early 2023, he joined Peking University as an Assistant Professor and Research Fellow at the Graduate School of Education.
Our team extends our sincere wishes for Dr. Fan’s success in embarking on his new endeavour and we look forward to maintaining a collaborative relationship with him.
Some notable publications authored by Dr. Fan during the FLoRA project include:
[1] Fan, Y., Lim, L., van der Graaf, J., Kilgour, J., Raković, M., Moore, J., … & Gašević, D. (2022). Improving the measurement of self-regulated learning using multi-channel data. Metacognition and Learning, 1-31.
[2] Fan, Y., van der Graaf, J., Lim, L., Raković, M., Singh, S., Kilgour, J., … & Gašević, D. (2022). Towards investigating the validity of measurement of self-regulated learning based on trace data. Metacognition and Learning, 17(3), 949-987.
[3] Fan, Y., Rakovic, M., van Der Graaf, J., Lim, L., Singh, S., Moore, J., … & Gašević, D. (2023). Towards a fuller picture: Triangulation and integration of the measurement of self‐regulated learning based on trace and think aloud data. Journal of Computer Assisted Learning.
[4] Srivastava, N., Fan, Y., Rakovic, M., Singh, S., Jovanovic, J., Van Der Graaf, J., … & Gasevic, D. (2022, March). Effects of internal and external conditions on strategies of self-regulated learning: A learning analytics study. In LAK22: 12th international learning analytics and knowledge conference (pp. 392-403).
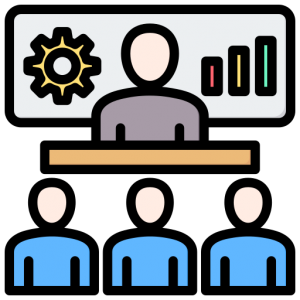
The Flora Team organised two workshops in the past few months to provide a platform for enthusiastic SRL researchers to examine existing self-regulated learning related work and further investigate how they can augment current techniques about instrumentation, measurement, analytics and feedback associated with SRL. Additionally, these workshops have facilitated (i) the commencement of inter-project level discussions aimed at promoting cross-team collaborations, and (ii) endowing the participants with practical opportunities for experiencing the assessment and promotion of SRL.
The first workshop was held during EC-TEL 2022, on the 13th September 2022, in Toulouse , France. A total of 16 participants attended our session. More details about the workshop can be accessed from: https://sites.google.com/monash.edu/ectel22-workshop-srl
Here are some pictures taken at the ECTEL 2022 workshop:
The second workshop was held during LAK2023 on the 14th March 2023, in Arlington, Texas, USA. A total of 27 participants attended our session. More details of the workshop can be accessed at: https://sites.google.com/monash.edu/lak23-trace-srl
Check out some pics from the LAK23 workshop:
Make sure to save our website link in order to stay informed about our forthcoming events.
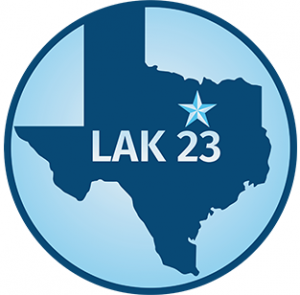
Our colleagues Dr. Mladen Rakovic , Dr Xinyu Li and Prof. Dragan Gasevic from the Monash University, Australia together with our collaborators Prof. Roger Azevedo, PhD students Megan and Daryn from University of Central Florida will be conducting an interactive workshop on measuring and facilitating self-regulated learning (SRL) at LAK 2023. More details can be obtained from the following website: https://sites.google.com/monash.edu/lak23-trace-srl
OVERVIEW
Prior research has shown that SRL skills are essential for successful life-long learning. Measuring SRL based on unobtrusive trace data and facilitating SRL based on real-time analysis. Such trace data have been pointed out as very valuable research directions. However, major challenges and significant gaps in this area are still many, such as i) the detection, measurement, and validation of SRL processes with trace data is still a much-debated issue within the SRL community; and ii) the design principles for effective interventions and the complex conditions and contexts when these interventions facilitated learning are still not known.
Therefore, we aim to improve the measurement and facilitation of SRL by hosting this full-day workshop, which will:
- initiate a project-to-project level dialogue to foster deep, cross-team collaborations and
- provide the participants with hands-on opportunities to experience the measurement and facilitation of SRL.
OBJECTIVES
From a research perspective, this workshop aims to: i) increase awareness of how tools and data channels can be combined to measure SRL; ii) elicit new approaches for detecting, measuring, and analysing SRL; iii) understand how combining student data and artificial intelligence can be used to create actionable insights into students’ learning; iv) design new representations/forms of communicating SRL scaffolding, dashboards, or feedback to facilitate learning.
From the participants’ perspective, we expect to: i) improve the knowledge and skills of participants about SRL measurement, learning processes, and SRL support; ii) produce a repository of new requirements, considerations and approaches of instruments for SRL; iii) build a research community, foster partnerships, and facilitate deployment of similar tools and analytics platforms as collaborative projects; and iv) explore opportunities for joint publications (e.g., a journal special issue), grants, and future workshops resulting from the collaborations.
More specifically, we have two objectives different from other workshops or research tracks in LAK23:
- Initiate a project-to-project level dialogue to foster deep, cross-team collaboration. By bringing together three of our SRL-related significant projects that measure and facilitate SRL following different analytic approaches, we aim to further promote research and practice that looks at SRL from a more comprehensive dimension of context, compared to single studies.
- Provide hands-on opportunity to experience the measuring and facilitating of SRL. Participants will also explore a learning analytic project and platform (developed and led by organizers, project name hidden for review) integrated with various instrumentation tools and personalised scaffoldings, and they will be able to explore their own multi-channel data and codesign possible SRL-related scaffoldings and feedback representations for learners and instructors.
Places are limited.
Registration for our workshop can be completed via the LAK 2023 website: https://www.solaresearch.org/events/lak/lak23/registration
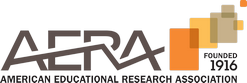
Our colleague, Dr. Yizhou Fan from the University of Edinburgh – UK, will be presenting our FLoRA work on the validity of measurement of SRL based on trace data at the AERA 2022 Symposium:
Presentation Title:
Toward Improving the Validity of Measurement of Self-Regulated Learning Based on Trace Data
Time: Fri, April 22, 11:30am to 1:00pm PDT | (Sat, April 23, 4:30 to 6:00am AEST
Description
Background. Contemporary research that looks at self-regulated learning (SRL) as processes of learning events derived from trace data has attracted an increasing interest over the past decade. Several researchers proposed trace-based measurement protocols of SRL processes which have been used in a series of subsequent studies (Siadaty et al., 2016, Saint et al., 2020, Fan et al., 2021). Because the use of trace data in measuring SRL is becoming more widespread, specific attention must be paid to whether trace data are reliable, and whether interpretations grounded in trace data are valid (Winne, 2020). However, limited research has been conducted that looks into the validity of trace-based measurement protocols.
Aims & Method. In order to fill this gap, we propose a novel validation approach (as shown in Figure 1) that combines theory-driven and data-driven perspectives to increase the validity of interpretations of SRL processes extracted from trace-data. We developed our own trace-based measurement protocols to translate raw trace data into SRL processes (such as Orientation and Monitoring); and we also used a previously developed coding scheme (Bannert, 2007) to code learners’ utterances into SRL processes. The hand-coded think-aloud data were used as “ground truth” for interpretation and validation of SRL processes derived from trace data. The main contribution of this approach consists of three alignments between trace data and think aloud data to improve measurement validity, see Figure 1. In addition, we define the match rate between SRL processes extracted from trace data and think aloud as a quantitative indicator to evaluate the “degree” of validity.
Findings & Significance. We tested this validation approach in a laboratory study that involved 44 learners who learned individually about the topic of artificial intelligence in education with the use of a technology-enhanced learning environment for 45 minutes. Following this new validation approach, we achieved an improved match rate between SRL processes extracted from trace-data and think aloud results (training set: 54.24%; testing set: 55.09%) compared to the match rate before applying the validation approach (training set: 38.97%; test set: 34.54%). By considering think-aloud data as “ground truth”, this improvement of the match rate quantified the extent to which validity can be improved by using our validation approach. It is also worth noting that, the shared theoretical background on SRL also played an essential role in informing the coding of think-aloud data and interpreting the SRL processes extracted from trace data. In conclusion, the novel validation approach presented in this study used both empirical evidence from think-aloud data and rationale from our theoretical framework of SRL, which now, allows testing and improvement of the validity of trace-based SRL measurements.
The full session details can be accessed via AERA 2022 Program Guide
Symposium Information :
Authenticating the Signal: Validating Digital Traces of Student Learning Using Concurrent, Corroborating Data Sources
Presenters in the session include:
Chair: Matthew Bernacki – University of North Carolina Chapel Hill
Discussant: Sanna Järvelä – University of Oulu
Presenting Authors: Matthew Graham (University of Oregon), Fatemeh Salehian Kia, (University of British Columbia), Matthew Graham (University of Oregon), Yizhou Fan (University of Edinburgh)
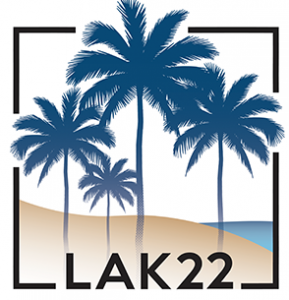
We are delighted to announce that we have two full papers accepted at LAK 22. LAK22 is organised by the Society for Learning Analytics Research (SoLAR) with location host University of California, Irvine. This year, the conference will be fully online from March 21-25, 2022.
Attend our sessions to know more about our work:
Full Paper:
[1] Srivastava, N., Fan , Y., Raković, M., Singh, S., Jovanovic, J., van der Graaf, J., Lim, L., Surendrannair, S., Kilgour, J., Molenaar, I., Bannert, M., Moore, J., and Gašević, D. (2022, March). Effects of Internal and External Conditions on Strategies of Self-regulated Learning: A Learning Analytics Study In Proceedings of the 12th International Conference on Learning Analytics & Knowledge (pp. TBA).
Abstract: Self-regulated learning (SRL) skills are essential for successful learning in a technology-enhanced learning environment. Learning Analytics techniques have shown a great potential in identifying and exploring SRL strategies from trace data in various learning environments. However, these strategies have been mainly identified through analysis of sequences of learning actions, and thus interpretation of the strategies is heavily task and context dependent. Further, little research has been done on the association of SRL strategies with different influencing factors or conditions. To address these gaps, we propose an analytic method for detecting SRL strategies from theoretically supported SRL processes and applied the method to a dataset collected from a multi-source writing task. The detected SRL strategies were explored in terms of their association with the learning outcome, internal conditions (prior-knowledge, metacognitive knowledge and motivation) and external conditions (scaffolding). The study results showed our analytic method successfully identified three theoretically meaningful SRL strategies. The study results revealed small effect size in the association between the internal conditions and the identified SRL strategies, but revealed a moderate effect size in the association between external conditions and the SRL strategy use.
[2] Raković, M., Fan, Y., van der Graaf, J., Singh, S., Kilgour, J., Lim, L., Moore, J., Bannert, M., Molenaar, I. and Gašević, D. (2022, March). Using Learner Trace Data to Understand Metacognitive Processes in Writing from Multiple Sources In Proceedings of the 12th International Conference on Learning Analytics & Knowledge (pp. TBA). Best Full Paper Nominee*
Abstract: Writing from multiple sources is a commonly administered learning task across educational levels and disciplines. In this task, learners are instructed to comprehend information from source documents and integrate it into a coherent written composition to fulfil the assignment requirements. Even though educationally potent, multi-source writing tasks are considered challenging to many learners, in particular because many learners underuse monitoring and control, critical metacognitive processes for productive engagement in multi-source writing. To understand these processes, we conducted a laboratory study involving 44 university students. They engaged in multi-source writing task hosted in digital learning environment. Adding to previous research, we unobtrusively measured metacognitive processes using learners’ trace data collected via multiple data channels and in both writing and reading space of the multi-source writing task. We further investigated how these processes affect the quality of a written product, i.e., essay score. In the analysis, we utilised both automatically and human-generated essay score. The rating performance of the essay scoring algorithm was comparable to that of human raters. Our results largely support the theoretical assumptions that engagement in metacognitive monitoring and control benefits the quality of written product. Moreover, our results can inform the development of analytics-based tools that support student writing by making use of trace data and automated essay scoring.
See you there!
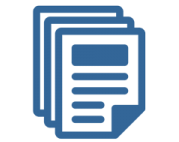
Our paper “Temporal Assessment of Self-Regulated Learning by Mining Students’ Think Aloud Protocols” has been recently published in the open-access journal Frontiers in Psychology (Section: Educational Psychology). We present major results of our research on self-regulated learning in online settings, as part of our FLoRA research project (ORA; DFG: BA2044/10-1; authors: Lyn Lim, Maria Bannert, Joep van der Graaf, Inge Molenaar, Yizhou Fan, Jonathan Kilgour, Johanna Moore, and Dragan Gašević).
Abstract: It has been widely theorized and empirically proven that self-regulated learning (SRL) is related to more desired learning outcomes, e.g., higher performance in transfer tests. Research has shifted to understanding the role of SRL during learning, such as the strategies and learning activities learners employ and engage in the different SRL phases which contribute to learning achievement. From a methodological perspective, measuring SRL using think aloud data has been shown to be more insightful than self-report surveys as it helps better in determining the link between SRL activities and learning achievements. Educational process mining on the basis of think aloud data enables a deeper understanding and more fine-grained analyses of SRL processes. Although students’ SRL is highly contextualized, there are consistent findings of the link between SRL activities and learning outcomes pointing to some consistency of the processes that support learning. However, past studies have utilized differing approaches which make generalization of findings between studies investigating the unfolding of SRL processes during learning a challenge. In the present study with 29 university students, we measured SRL via concurrent think aloud protocols in a pre-post design using a similar approach from a previous study in an online learning environment during a 45-minute learning session where students learned about three topics and wrote an essay. Results revealed significant learning gain and replication of links between SRL activities and transfer performance, similar to past research. Additionally, temporal structures of successful and less successful students indicated meaningful differences associated with both theoretical assumptions and past research findings. In conclusion, extending prior research by exploring SRL patterns in an online learning setting provides insights to the replicability of previous findings from online learning settings and new findings show that it is important not only to focus on the repertoire of SRL strategies but also on how and when they are used.
Lim, L., Bannert, M., van der Graaf, J., Molenaar, I., Fan, Y., Kilgour, J., Moore, J., & Gašević, D. (2021). Temporal Assessment of Self-Regulated Learning by Mining Students’ Think-Aloud Protocols. Frontiers in Psychology, 12, 5122.
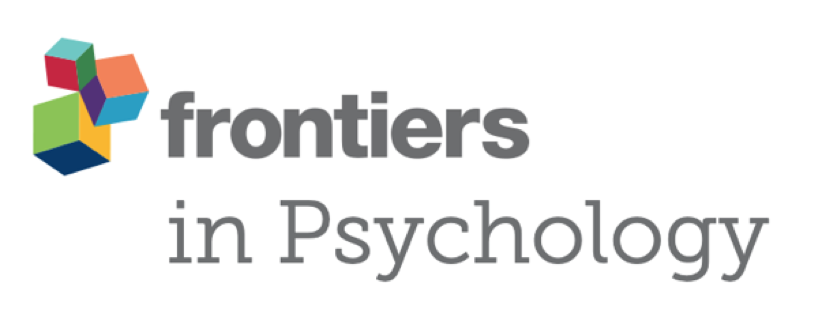
The paper can be viewed and downloaded here: https://doi.org/10.3389/fpsyg.2021.749749
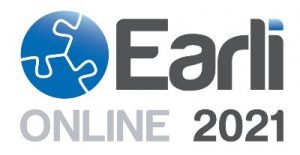
We hold our inaugural FLoRA symposium: “New Ways of Measuring, Analysing and Scaffolding Self-Regulated Learning” at the 19 th Biennial European Association for Research on Learning and Instruction – EARLI 2021 conference (link: https://earli.org/EARLI2021). In light of the COVID-19 pandemic, the EARLI 2021 conference will take place online with the conference theme – Education and Citizenship: learning and Instruction and the Shaping of Futures. We look forward to seeing you at the conference!
Besides, Prof. Maria Bannert will also join the other members of the EARLI-Centre for Innovative Research (E-CIR), “Measuring and Supporting Student’s Self-Regulated Learning in Adaptive Educational Technologies“, in an expert panel discussion. In light of the COVID-19 pandemic, the EARLI 2021 conference will take place online with the conference theme – Education and Citizenship: learning and Instruction and the Shaping of Futures.
The contributions are as follows:
Project FLoRA EARLI Symposium: New Ways of Measuring, Analysing and Scaffolding Self-Regulated Learning
Organizer: Joep van der Graaf, Radboud University, Netherlands
Discussant: Philip Winne, Simon Fraser University, Canada
Date & Time: 26 Aug 2021, 15:45 – 16:45
Location: Session Room 5 – Session T
New ways of measuring and analysing Self-Regulated Learning (SRL) are rapidly emerging. This has important implications for theoretical frameworks of SRL, methodological approaches, and for current educational practices. The first aim of this symposium is to present and discuss new approaches to measurement and analysis of SRL. The second aim is to provide suggestions on the design of educational materials that provide additional insights into students’ learning processes and support their SRL. The presenters are a group of international researchers, who have a strong interest in learning analytics and/or SRL. The presentations are incrementally ordered, moving from measurement and analysis of SRL (1, 2) to learning outcomes (3) and ending with a digital learning tool (4). The four presentations revolve around the following main questions: a) How can multimodal data improve the granularity of measurement of SRL? b) How does SRL unfold in high versus low performing students? c) How do SRL activities relate to different learning outcomes? And d) How can we support students by visualising their SRL process? By addressing these questions, this symposium provides suggestions for theory and methodological development as well as educational practice.
[1] Improving the granularity for the measurement of self-regulated learning using multi-channel data.
Yizhou Fan, The University of Edinburgh, United Kingdom; Lyn Lim, Technical University of Munich, Germany; Joep van der Graaf, Radboud University, Netherlands; Jonathan Kilgour, The University of Edinburgh, United Kingdom; Johanna Moore, The University of Edinburgh, United Kingdom; Dragan Gasevic, Monash University, Australia; Maria Bannert, Technical University of Munich, Germany; Inge Molenaar, Radboud University, Netherlands.
In recent years, unobtrusive measures of self-regulated learning (SRL) processes based on log data recorded by digital learning environments have attracted increasing attention. However, researchers have also recognised that simple navigational log data or time spent on pages are often not fine-grained enough to study complex SRL processes. Recent advances in data-capturing technologies enabled researchers to go beyond simple navigational logs to measure SRL processes with multi- channel data. Though, to what extent can the addition of peripheral and eye-tracking data with navigational data improve the granularity of measurement of SRL are key questions that require further investigation. Hence, we conducted a study that aimed to address this problem by enhancing navigational log data with peripheral and eye-tracking data. Based on the measurement protocol proposed in this study, we were able to compare the process models of SRL of n=25 students across different data channels. The results revealed that by adding new data channels, we improved the capture of learning actions and detected SRL processes while enhancing the granularity of the measurement. In addition, we also concluded that eye-tracking data is valuable for measuring and extracting SRL processes, and it should receive more attention in the future.
[2] Understanding Self-Regulated Learning Processes through Process Mining.
Lyn Lim, Technical University of Munich, Germany; Maria Bannert, Technical University of Munich, Germany; Joep van der Graaf, Radboud University, Netherlands; Yizhou Fan, The University of Edinburgh, United Kingdom; Jonathan Kilgour, The University of Edinburgh, United Kingdom; Inge Molenaar, Radboud University, Netherlands; Johanna Moore, The University of Edinburgh, United Kingdom; Dragan Gasevic, Monash University, Australia.
Self-regulated learning (SRL) is related to better learning outcomes and observation of SRL using think aloud data has been shown to be more insightful in determining SRL activities and predicting students’ learning achievements than self-reports. Educational process mining, moreover with think aloud data, enables a deeper understanding and a more fine-grained analysis of SRL processes. This study based on a pre-post design aimed to investigate how students differ in SRL learning processes and how this affects learning performance. There were 32 university students who participated in the study to learn about the theme, “Artificial Intelligence in Education”, and they had to write an essay in a digital learning environment within a 45-minute learning session while thinking aloud. The results showed that there is a significant learning gain in the knowledge test. Besides, the top performers showed more metacognitive and cognitive activities during learning. Furthermore, process mining using HeuristicMiner algorithm based on post hoc coded think aloud protocols examined differences in process structures of SRL for the high and low performers. In general, comparing resulting process mining models with prior process mining models will help to better generalize findings of prior research.
[3] How Self-Regulated Learning Affects Different Learning Outcomes.
Joep van der Graaf, Radboud University, Netherlands; Lyn Lim, Technical University of Munich, Germany; Yizhou Fan, The University of Edinburgh, United Kingdom; Jonathan Kilgour, The University of Edinburgh, United Kingdom; Johanna Moore, The University of Edinburgh, United Kingdom; Dragan Gasevic, Monash University, Australia; Maria Bannert, Technical University of Munich, Germany; Inge Molenaar, Radboud University, Netherlands.
Self-regulated learning (SRL) fosters transfer, but effects on other learning outcomes, such as domain knowledge are mixed. SRL potentially has a differential impact on learning outcomes with different characteristics, deep vs surface knowledge, and independent vs connected concepts. Therefore, we assessed how surface knowledge measured with a domain test (independent), and a concept map (connected) and deep knowledge measured with a transfer test (independent) and an essay (connected) are associated to SRL activities during learning and to prior metacognitive knowledge. Forty-five university students performed a 45 minute problem-solving task integrating three topics into a vision on future of education. SRL activities were measured using think aloud. Results revealed learning occurred. Surface knowledge measures, independent and connected concepts, were related to each other and associated with low cognitive activities during learning. Deep knowledge of independent concepts was associated with low cognitive processes, while deep knowledge of connected concepts was associated with a mixture of low and high cognitive processes. In addition, we found that prior metacognitive knowledge was associated with deep knowledge of independent concepts. To conclude, taking the level and structure of knowledge into account helps to specify effects of SRL processes on learning outcomes.
[4] Visualising student’s learning strategies in online learning to support self-regulation.
Shaveen Singh, Monash University, Australia; Mladen Rakovic, Monash University, Australia, Yizhou Fan, The University of Edinburgh, United Kingdom; Lyn Lim, Technical University of Munich, Germany; Joep van der Graaf, Radboud University, Netherlands; Jonathan Kilgour, The University of Edinburgh, United Kingdom; Inge Molenaar, Radboud University, Netherlands; Johanna Moore, The University of Edinburgh, United Kingdom; Maria Bannert, Technical University of Munich, Germany; Dragan Gasevic, Monash University, Australia.
Visualisations provide an effective way for learners to gain insight into their learning process which, in turn, may promote their self-regulated learning. Yet few learner-facing visualisations have been developed to support learners’ self-regulation. To this purpose, we propose a collection of personalised, theory-based and empirically driven visual interfaces. We harnessed trace data from multiple channels to generate clear and actionable recommendations for learners to improve their regulation. Guided by a quasi-experimental study in an university context (n=25), we investigated the student’s critical learning processes in SRL, such as, planning, content consumption, working on task, monitoring and evaluation. In the presentation, we describe the learning environment to collect data about those processes, and suggest visualizations that rely upon these data sources. In an ongoing study, we will prompt learners to engage in metacognitive monitoring of their learning using visualisations to support their regulation and learning.
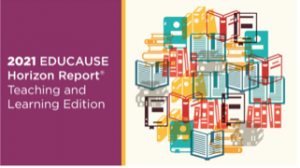
FLoRA-Project featured in the EDUCAUSE 2021 Horizon Report
Our ORA research project, FLoRA – Facilitating Self-Regulated Learning with Personalized Scaffolds on Student’s own Regulation Activities (funded by the DFG, ESRC, NWO) was selected to be featured in the EDUCAUSE 2021 Horizon Report | Teaching and Learning Edition.
The EDUCAUSE 2021 Horizon Report profiles key trends and emerging technologies and practices shaping the future of teaching and learning and envisions a number of scenarios and implications for that future. It is based on the perspectives and expertise of a global panel of leaders from across the higher education landscape. Six out of 141 key technologies and practices were voted to have a significant impact on the future of higher education teaching and learning. The report serves as one vantage point on where our post-pandemic future of teaching and learning may be headed.
The report was officially published on 26 April and can be downloaded from here:
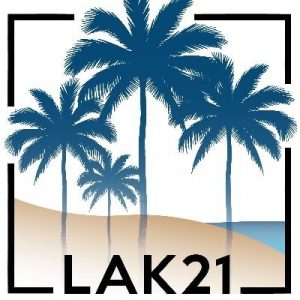
We are delighted to be presenting our work at LAK 21. LAK21 is organised by the Society for Learning Analytics Research (SoLAR) with location host University of California, Irvine. This year, the conference will be fully online from April 12-16, 2021.
Attend our sessions to know more about our work:
Full Paper:
[1] van der Graaf, J., Molenaar, Lim L., I., Fan, Y.,Kilgour, J., Moore, J., Dasevic, D. & Bannert, M. (2021, April). Do Instrumentation Tools Capture Self-Regulated Learning? In Proceedings of the 11th International Conference on Learning Analytics & Knowledge (pp. 438-448).
Abstract: Researchers have been struggling with the measurement of SelfRegulated Learning (SRL) for decades. Instrumentation tools have been proposed to help capture SRL processes that are difficult to capture. The aim of the present study was to improve the measurement of SRL by embedding instrumentation tools in a learning environment and validating the measurement of SRL with these instrumentation tools using think aloud. Synchronizing log data and concurrent think-aloud data helped identify which SRL processes were captured by particular instrumentation tools. One tool was associated with a single SRL process: the timer co-occurred with monitoring. Other tools co-occurred with a number of SRL processes, i.e., the highlighter and note-taker captured superficial writing down, organizing, and monitoring, whereas the search and planner tools revealed planning and monitoring. When specific learner actions with the tool were analyzed, a clearer picture emerged of the relation between the highlighter and note taker and SRL processes. By aligning log data with think-aloud data, we showed that instrumentation tool use indeed reflects SRL processes.
The main contribution is that this paper is the first to show that SRL processes that are difficult to measure by trace data can indeed be captured by instrumentation tools such as high cognition and metacognition. Future challenges are to collect and process log data in real-time with learning analytic techniques to measure ongoing SRL processes and support learners during learning with personalized SRL scaffolds.
Poster:
[2] Singh, S., Rakovic, M., Lim, K.P., van der Graaf, J., Fan, Y.,Kilgour, J., Bannert, M., Molenaar, I., Gasevic, D. & Moore, J. (2021, April). Using Enhanced Learner-facing Visual Interfaces to support Self-regulated Learning. In Companion Proceedings of the 11th International Conference on Learning Analytics & Knowledge (pp. 661-63).
Abstract: Visualisations provide a rapid way for learners to see and understand their learning metrics. Yet few learner-facing interfaces have been developed to support learners’ self-regulation. This paper proposes the application profile of personalised visual interfaces to support learners in self-regulated learning (SRL). Our design is theoretically based and empirically driven, and utilises trace data from multiple channels to provide clear actionable recommendations for learners to improve regulation. Guided by a quasi-experimental study in a university context, we survey the critical learning processes in SRL, describe the environment to collect multimodal and multichannel data about those processes, and suggest visualizations that can rely upon these data sources— to prompt learners to engage in metacognitive monitoring of their learning and adapt their learning. We conclude with future directions of contributing to the interdisciplinary efforts of addressing the use of cognitive and meta-cognitive data traces and visualisations to foster self-regulation to support optimal and successful learning.
See you there !